Posted on Thursday, April 22, 2021
This session is jointly sponsored by the Statistics department and the Research
Methods, Measurement, and Evaluation program, University of Connecticut
(UCONN), New England Statistical Society (NESS) and Statistical and Applied
Mathematical Institute (SAMSI) as part of online interdisciplinary seminar
series on statistical methodology for social and behavior research.
Speaker: DR. JENNIFER HILL, NEW YORK UNIVERSITY
Date and Time: FRIDAY, 4/30/2021, 12PM
Topic: THINKCAUSAL: ONE STOP SHOPPING FOR ANSWERING YOUR CAUSAL INFERENCE
QUESTIONS
Abstract:
Causal inference is a necessary tool in education research for answering
pressing and ever-evolving questions around policy and practice. Increasingly,
researchers are using more complicated machine learning algorithms to estimate
causal effects. These methods take some of the guesswork out of analyses,
decrease the opportunity for “p-hacking,” and are often better suited for
more fine-tuned causal inference tasks such as identifying varying treatment
effects and generalizing results from one population to another. However, these
more sophisticated methods are more difficult to understand and are often only
accessible in more technical, less user-friendly software packages. The
thinkCausal project is working to address these challenges (and more) by
developing a highly scaffolded multi-purpose causal inference software package
with the BART predictive algorithm as a foundation. The software will scaffold
the researcher through the data analytic process and provide options to access
technology-based teaching tools to understand foundational concepts in causal
inference and machine learning. This talk will briefly review BART for causal
inference and then discuss the challenges and opportunities in building this
type of tool. This is work in progress and the goal is to create a conversation
about the tool and role of education in data analysis software more broadly.
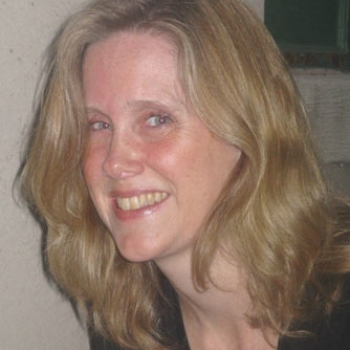
Bio: Dr. Jennifer Hill develops and evaluates methods that help us answer the
causal questions that are vital to policy research and scientific development.
In particular she focuses on situations in which it is difficult or impossible
to perform traditional randomized experiments, or when even seemingly pristine
study designs are complicated by missing data or hierarchically structured
data. Most recently Hill has been pursuing two intersecting strands of
research. The first focuses on Bayesian nonparametric methods that allow for
flexible estimation of causal models and are less time-consuming and more
precise than competing methods (e.g. propensity score approaches). The second
line of work pursues strategies for exploring the impact of violations of
typical causal inference assumptions such as ignorability (all confounders
measured) and common support (overlap). Hill has published in a variety of
leading journals including Journal of the American Statistical Association,
Statistical Science, American Political Science Review, American Journal of
Public Health, and Developmental Psychology. Hill earned her PhD in Statistics
at Harvard University in 2000 and completed a postdoctoral fellowship in Child
and Family Policy at Columbia University’s School of Social Work in 2002. Hill
is also the Director of the Center for Practice and Research at the
Intersection of Information, Society, and Methodology (PRIISM) and Co-Director
of and the Master of Science Program in Applied Statistics for Social Science
Research (A3SR).
For inquiry, please contact Dr. Xiaojing Wang at xiaojing.wang@uconn.edu