Posted on Tuesday, May 18, 2021
This session is jointly sponsored by the Statistics department and the Research
Methods, Measurement, and Evaluation program, University of Connecticut
(UCONN), New England Statistical Society (NESS) and Statistical and Applied
Mathematical Institute (SAMSI) as part of online interdisciplinary seminar
series on statistical methodology for social and behavior research.
Speaker: DR.David Kaplan, University of Wisconsin – Madison
Date and Time: FRIDAY, 5/21/2021, 12PM
Topic: Developments and Extensions in the Quantification of Model
Uncertainty: A Bayesian Perspective
Abstract:
Issues of model selection have dominated the theoretical and applied
statistical literature for decades. Model selection methods such as ridge
regression, the lasso and the elastic net have replaced ad hoc methods such as
stepwise regression as a means of model selection. In the end, however, these
methods lead to a single final model that is often taken to be the model
considered ahead of time, thus ignoring the uncertainty inherent in the search
for a final model. One method that has enjoyed a long history of theoretical
developments and substantive applications, and that accounts directly for
uncertainty in model selection, is Bayesian model averaging (BMA). BMA
addresses the problem of model selection by not selecting a final model, but
rather by averaging over a space of possible models that could have
generated the data. The purpose of this paper is to provide a detailed and
up-to-date review of BMA with a focus on its foundations in Bayesian
decision theory and Bayesian predictive modeling. We consider the selection
of parameter and model priors as well as methods for evaluating predictions
based on BMA. We also consider important assumptions regarding BMA and
extensions of model averaging methods to address these assumptions,
particularly the method of Bayesian stacking. Extensions to problems of
missing data and probabilistic forecasting in large-scale educational
assessments are discussed.
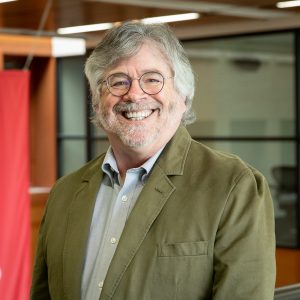
Bio: Dr. David Kaplan is the Patricia Busk Professor of Quantitative Methods in the Department of Educational Psychology at the University of Wisconsin – Madison. His research focuses on the development of Bayesian statistical methods for education research, with applications to large-scale cross-sectional and longitudinal survey designs. Dr. Kaplan is an elected member of the National Academy of Education and serves as the chair of its Research Advisory Committee; a recipient of the Samuel J. Messick Distinguished Scientific Contributions Award from the American Psychological Association (Division 5); a past-President of the Society for Multivariate Experimental Psychology; a fellow of the American Psychological Association (Division 5); a recipient of the Alexander Von Humboldt Research Award; an Honorary Research Fellow in the Department of Education at the University of Oxford; and a fellow of the Leibniz Institute for Educational Research and Information and the Leibniz Institute for Educational Trajectories. He was also a Jeanne Griffith Fellow at the National Center for Education Statistics. He received his Ph.D. in Education from UCLA in 1987.
For inquiry, please contact Dr. Xiaojing Wang at xiaojing.wang@uconn.edu